Implementing AI in the banking and insurance sectors isn’t just about innovation – it’s about responsibility and adherence to evolving regulations. As the financial service sector faces tightening regulatory frameworks and AI technologies grow more complex, financial service providers must adopt a compliance-driven strategy to ensure trust, transparency, and accountability.
This article outlines a four-part framework for building such a strategy, beginning with establishing ethical AI governance, integrating AI into existing compliance systems, ensuring explainability and accountability in decision-making, and empowering teams with the right skills and mindset. Each component is key to creating AI systems that not only work but comply with regulatory standards.
Component 1: Develop an ethical AI governance framework
The first step for both, insurers and banks, venturing into AI is to establish clear governance around AI systems. This means defining the principles, policies, and processes that will apply to AI systems from development through deployment. An ethical AI framework typically covers areas like fairness (preventing bias), transparency, data privacy, security, and accountability. It should align with industry best practices and regulations
For example, regulations such as the EU AI Act impose clear guidelines for high-risk AI applications in the financial and insurance sectors, and AI governance frameworks should be designed accordingly. Additionally, banks and insurers must implement internal protocols for AI risk management, including impact assessments (e.g. Data Protection Impact Assessments for GDPR or bias audits) and regular validation of AI model performance.
The governance framework should also assign ownership, such as forming an AI governance committee or assigning a Chief AI Ethics Officer, to ensure there is accountability at the top for how AI is used. By developing this framework early, financial institutions set the tone that AI will be deployed responsibly. In fact, regulators view this favorably. Demonstrating that you have a “responsible AI” program in place goes a long way in proving that your organization is taking compliance seriously.
Ultimately, a sound AI governance framework is essential for a well-functioning, trusted and compliant financial service sector, providing the foundation upon which all other AI efforts are built upon.
Component 2: Integrate AI with existing compliance systems and processes.
AI solutions should not function as standalone systems. To ensure their full potential is realized, AI must be integrated seamlessly into existing compliance frameworks. For both banks and insurers, this integration involves connecting AI tools with established workflows and legacy systems to ensure that AI-driven insights are incorporated into day-to-day operations.
On the technical side, an AI compliance tool (such as machine learning models for fraud detection or compliance monitoring) should feed its outputs into the same dashboards or case management systems that compliance officers already use. For instance, an AI-powered transaction screening tool might flag suspicious activities, which then gets automatically logged in a shared compliance platform. This integration allows compliance officers to review AI-generated insights alongside their usual data, improving efficiency and accuracy. AI can also be integrated by connecting to data sources across the company – policy admin systems, claim systems – to draw a comprehensive picture for compliance monitoring. Modern AI “agents” are designed to plug into existing workflows, rather than replace them wholesale.
For example, an AI agent might automatically review documents for compliance errors and then update a compliance checklist that staff currently maintain, effectively automating one step in an existing process. Procedurally, integration means updating compliance team protocols to include AI insights: if AI detects an anomaly, what is the escalation path? How do humans verify AI findings? Those questions should be answered in the compliance playbooks.
AI can also reduce operational friction by automating tasks like regulatory change tracking, enabling the team to focus on more critical analysis. Over time, these integrations create a unified compliance ecosystem where AI proactively supports compliance efforts, and human judgment ensures that the rules are followed.
This ensures that human expertise and AI insights complement each other. The goal is a seamless process where AI handles the heavy data crunching and preliminary analysis, and then human compliance professionals use that information to make informed decisions. This synergy improves both efficiency and control. Over time, these integrations will lead to a more unified compliance ecosystem where AI proactively does the ground work and humans provide oversight and final judgement.
Navigating compliance is complex—your AI strategy shouldn’t be.
Discover how to build ethical, explainable, and accountable AI that helps you meet regulations and stay compliant.
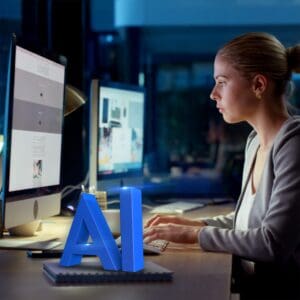
Component 3: Ensure explainability and accountability in AI decision-making
Incorporating AI into compliance does not remove the need for accountability and explainability – in fact, it heightens it. Regulators will ask, “Who is responsible for decisions made by AI?” and “Can you show why the AI made that choice?” The questions seem to be simple but the answer might lead to difficulties in reality. Generally speaking, every AI system deployed must be explainable and decisions must be traceable.
AI systems, such as those used for negative news screening in banks or claims processing in insurance, should include mechanisms to explain decision-making in a manner understandable to humans. For instance, an AI model that denies a claim should be able to provide a clear rationale for its decision, including key data points that influenced the outcome.
Additionally, audit trails and logging for AI systems should be put into place – the AI should log its actions and decisions in a way that can be reviewed after the fact. Many AI platforms now provide an “audit log” feature out of the box.
On the accountability front, there should be a clear chain of responsibility with designated owners for each AI application. This ensures there is always a human who can answer for the AI’s behavior and intervene if necessary.
Regular model reviews—like model risk management in banking—ensure that AI models continue to perform in compliance with regulatory standards. In case of discrepancies or errors, a clear chain of accountability should be in place, designating responsible personnel for each AI-driven decision. This is often a joint effort between the data science team and the risk/compliance team.
Strong corporate governance is needed to oversee AI outcomes – meaning senior management, and possibly the board, should get periodic reports on how AI models are performing, any incidents or overrides, and any emerging risks. By enforcing explainability and clear accountability, financial service providers can use AI as a trustworthy aid rather than a “black box.” This not only satisfies regulators (who are increasingly asking for transparency and proof of oversight in AI use), but also makes internal stakeholders more comfortable with AI-driven processes. Remember, the aim is to have AI decisions that can be understood, justified, and, if needed, challenged or corrected by humans – achieving that is a cornerstone of a compliance-first AI strategy.
Component 4: Train and empower teams to manage AI-driven compliance:
The best technology will fall short if the people using it are not prepared. As financial service providers adopt AI in compliance, they must invest in developing the skills and knowledge of their teams. This involves cross-functional training: compliance officers need to become conversant with data analytics and AI concepts, while data scientists and IT staff need to understand regulatory requirements and the compliance context. Specifically, compliance and risk teams should be trained on how to interpret AI outputs, how to handle AI-generated alerts, and how to oversee AI systems.
Meanwhile, AI developers should be educated on privacy laws, ethical AI principles, and what regulators expect. Identifying skill gaps is a good starting point – for example, does your compliance department have someone who understands AI model bias, or your IT team someone who knows cybersecurity implications of AI? Many organizations find gaps in data literacy, AI ethics and governance, and overall digital fluency on their teams. Targeted workshops and courses can address these. Some financial service institutions are appointing “AI champions” within departments – individuals with hybrid expertise who can bridge the gap between technical teams and compliance teams.
Encouraging a collaborative mindset is also important. Encourage teams to view AI as a support tool, not a threat to their jobs. Scenario-based training—where teams practice investigating AI-triggered alerts or audit AI decisions—ensures that employees are prepared to handle AI-driven processes responsibly. By empowering employees with the right skills and a clear understanding of AI’s role, organizations ensure that their AI-driven compliance processes are effectively managed and continuously improved. In fact, a culture of continuous learning around AI will keep the organization adaptable as AI technologies and regulations evolve.
Final thoughts
At Eraneos, we recognize that marrying AI innovation with strict regulatory compliance is a delicate balance – especially in industries as highly regulated as banking and insurance. Our approach is rooted in deep industry expertise combined with cutting-edge technological know-how. We have a team of seasoned professionals with in-depth understanding of risk management and regulatory compliance across the financial service sector. This includes legal and compliance experts who stay abreast of the latest regulations, as well as data scientists and AI specialists experienced in building solutions for highly regulated environments.
By bringing these skill sets together, we help our clients design AI strategies and solutions that are compliant by design. From the outset of any AI initiative, we work with our clients to incorporate robust governance and risk management measures – essentially, we help you build the “guardrails” before hitting the gas on AI.
Incorporating advanced AI techniques such as sentiment analysis using web crawling, dynamic clustering to identify high-risk groups, and machine learning models to reduce false positives, we help our clients gain a deeper, actionable understanding of regulatory data and compliance risk. Through responsible AI deployment and continuous learning, we ensure our clients remain compliant while reaping the full benefits of AI-driven innovation.
We ensure that principles like fairness, accountability, and transparency are baked into your AI projects, for example, by defining clear guidelines for model development and validation, documentation requirements, escalation procedures for AI decisions. With Eraneos, you’re not just implementing AI – you’re implementing AI responsibly.