As organizations increasingly strive for data-driven decision making, improving data quality is a logical first step. Checking fields, validating reference lists, and cleansing records have become commonplace. But those who think this is enough for an organization to rely on data are only looking at the tip of the iceberg. Data quality is an important foundation, but not an end in itself. The real work begins when organizations embrace information quality and actively build trust in the data they use.
Data quality ≠ Information quality
There is no single definition of data quality, but there is a general rule of thumb. Your data is high quality if it is suitable for use in a particular context. Well-known data quality dimensions such as accuracy, completeness, consistency, and timeliness are described in the DAMA-DMBoK. These are helpful as guidelines, but they do not tell you anything about the reliability of information in the context of a strategic decision.
While data quality is often limited to individual data points, such as entering a date of birth or zip code, information quality is about the coherence and meaning of that data. Is the combination of data logical and usable within the intended process or report? That’s where the difference begins.
From control to context
Many organizations take a highly technical approach. Scripts check fields, validations run in the background, and dashboards monitor anomalies. Definitely a good foundation! But this approach often misses the context in which data is used.
A field can be correct on its own, but still lead to a wrong conclusion if it does not fit into the context of the business process. Consider a date of birth that is correct, but combined with an incorrect contract type results in an incorrect customer profile. In this case, the problem is not data quality, but information quality. Information quality requires more than just technical checks. It requires understanding the meaning of the data in a broader context and translating the underlying business logic.
Business rules as a starting point
Organizations that truly want to work data-driven and monitor their business using reliable information do not start by checking data, but by formulating the information needed. What is the question to be answered? What definitions are relevant? And which data attributes are important? From this need, business rules should be created: rules that describe when information can be considered correct and reliable. These rules often go beyond traditional validation because they include context and exceptions. For example, validating a housing contract for seniors requires not only a date of birth, but also an understanding of the type of contract, temporary arrangements, and residing relatives. Only by including all of these elements does a reliable picture emerge.
DAMA-DMBoK
The DAMA-DMBoK provides a useful set of dimensions for structuring data quality, but it is not intended to be a strict blueprint. In practice, the classification sometimes proves to be artificial: quality problems do not always fit neatly into one dimension. The ultimate question is not which dimension a problem falls into, but whether the data is fit for purpose. Even data that meets all the technical requirements can be perceived as unreliable if it does not fit the overall picture. In this respect, information quality is as much a matter of perception as it is of technology. Users need to trust it, and that trust only comes when the context is right.
Ready to transform your data quality into trusted, actionable insights?
Discover how our structured data management framework can help you get there.
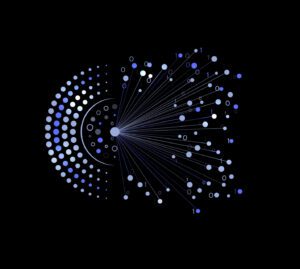
Trust is not an output of tooling
There are many tools available for monitoring and optimizing data quality. But tools are tools, not magic wands. They do not automatically solve the underlying trust in information. Trust only comes when data is understandable, traceable and actionable. When people understand what numbers mean, how they are constructed, and why they are correct or not. Tools support this process, for example, when data is spread across multiple systems or when traditional DQ initiatives fall short. But they can only be effective if the information needs are clear, and the full context is included. This requires communication, transparency and collaboration, not more validation rules.
People will trust the data as information if they are told by trusted colleagues that it is accurate, if their questions are well answered, if more and more people are using it and saying positive things about it, if they are involved in it, and if it is published.
Information that actually makes a difference
Directors don’t want to see validation reports or technical scores. They want to know: Can I trust these numbers? Is the information I have in front of me complete, accurate, and useful for the decision I need to make? Turning data into trusted information requires collaboration between data experts and business stakeholders. It’s about building a common understanding: this is what the data says, this is why it’s right, and this is what we can do with it. Once that common language is in place, there is room for trust. And that trust is what the organization needs to steer with confidence.
From pitches to confidence
Data quality is indispensable. But organizations that want to be data-driven cannot stop there. Information quality and especially trust in it is what ultimately matters. That requires a shift from technical checks to contextual insight. From checklists to business rules and from data to meaningful information. Only when data is understood, explained and trusted does it create the space for data-driven decision-making with impact.